AI-Powered Childcare: Ushering in a New Era of Support, Safety & Smart Parenting
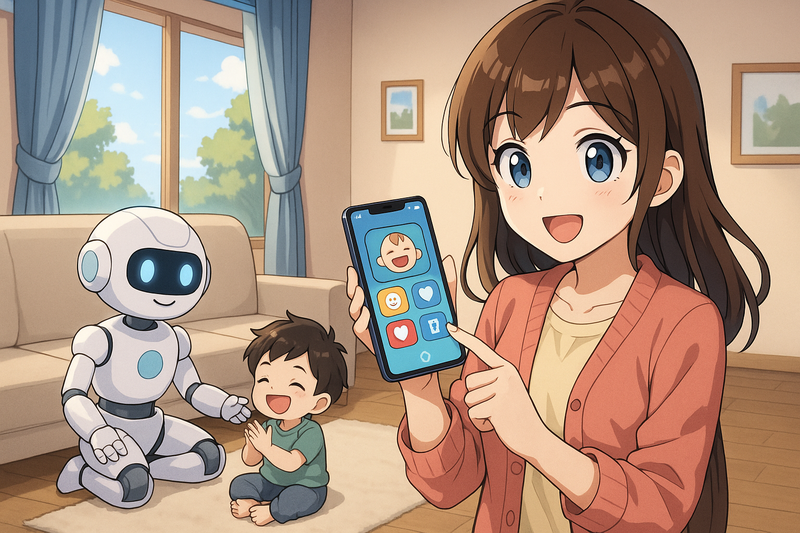
Discover how AI is transforming childcare—enhancing safety, education & personalized parenting. A smarter future starts here.
Introduction: The Dawn of AI in Childcare
Technology's integration into daily life continues to accelerate, reshaping industries from finance to healthcare. Early childhood education and care (ECEC) is no exception, witnessing a shift from basic digital tools towards more sophisticated applications driven by artificial intelligence (AI). This evolution sparks both excitement and critical reflection, as AI promises unprecedented opportunities while demanding careful consideration of its impact on our youngest learners and their caregivers.
The potential of AI in the childcare landscape appears multifaceted, offering a triple promise centered around three core pillars:
- Supporting Child Development: AI tools show potential for enhancing early developmental monitoring, enabling the detection of subtle patterns or potential risks much earlier than traditional methods might allow, thereby facilitating timely intervention.
- Enhancing Learning: AI-driven educational platforms can offer personalized learning experiences, adapting to individual paces and styles, potentially boosting cognitive skills and engagement.
- Aiding Caregivers: AI applications aim to alleviate the significant administrative burden faced by childcare professionals and parents, automating tasks and providing personalized support to help mitigate burnout and free up time for meaningful interaction.
While the allure of these advancements is strong, it is crucial to approach this new era with a balanced perspective. The integration of AI into the sensitive realm of childcare necessitates responsible innovation, rigorous ethical scrutiny, and an unwavering commitment to preserving the essential human element in care and education. Initial reports and queries often highlight specific, high-impact claims – such as suggestions of 90% accuracy in milestone prediction by Stanford research or a 15% cognitive gain from apps cited in Nature. This blog post aims to provide an evidence-based overview by delving into the broader evidence and specific, verifiable findings from a range of research, including work involving Stanford researchers and studies published in reputable journals, to paint a nuanced picture of AI's emerging role. It will explore the capabilities, documented benefits, underlying technologies, and critical ethical considerations shaping the future of AI-powered childcare.
Section 1: AI as a Partner in Monitoring Early Development
The Criticality of Early Insights
The first few years of life are a period of rapid and foundational development across motor, cognitive, social, and emotional domains. Monitoring this development is paramount, as early identification of potential delays or risks allows for timely interventions, which are known to significantly improve long-term outcomes. Traditionally, developmental monitoring relies heavily on parental reports, caregiver observations, and periodic screenings during well-child visits. While valuable, these methods can be subjective, infrequent, and may miss subtle signs that emerge between scheduled check-ups. AI presents a potential paradigm shift, offering tools for more continuous, objective, and data-driven developmental surveillance.
How AI "Sees" Development
AI systems leverage sophisticated techniques to gather and interpret developmental data, often focusing on observable behaviors like movement, which is a critical indicator in early infancy.
- Movement Analysis: AI algorithms can process movement data captured through various means. This includes specialized equipment like 3D motion capture systems (e.g., Vicon) used in research settings , wearable sensors embedded in clothing (like jumpsuits with inertial measurement units) , or even video footage captured by standard smartphone cameras. These systems track the motion of different body parts, analyzing patterns, frequency, quality, and symmetry of movements over time.
- AI Techniques: Machine learning (ML) and deep learning (DL) models form the core of this analysis. Simpler ML classifiers like decision trees, k-nearest neighbors (k-NN), and support vector machines (SVMs) have been used to classify infants based on movement recordings and demographic data. More complex DL models, such as Convolutional Neural Networks (CNNs) and specialized architectures like 2D-CapsNet , are employed to analyze nuanced patterns in movement data, classify different types of actions (e.g., spontaneous vs. purposeful), identify developmental stages or milestones, and detect subtle anomalies or asymmetries that might indicate developmental differences.
This technological approach moves beyond simple observation towards quantifiable analysis. By processing vast amounts of movement data, AI can potentially identify patterns invisible to the human eye or patterns that only become apparent over extended periods, offering a more granular view of an infant's developmental trajectory. This objective, data-driven approach holds the potential to complement traditional assessment methods, providing richer insights for parents and professionals.
Accuracy and Findings
Research exploring AI's capabilities in developmental monitoring has yielded promising results regarding accuracy, offering context to initial claims about high prediction rates. Key findings include:
- Predicting Outcomes: In a study involving infants at risk (AR) for developmental delays, AI models using day-long inertial motion recordings and demographic data achieved an 89.5% overall accuracy in predicting future developmental outcomes. This underscores AI's potential not just for classification but for prognostication, which is vital for targeting early interventions effectively.
- Classifying Movement Stages: A deep learning model, 2D-CapsNet, demonstrated 86% accuracy when analyzing only foot movements to classify infants' engagement across different stages of an experimental interaction with a mobile. This high accuracy, based on a specific body part, highlights AI's sensitivity in detecting subtle behavioral shifts related to learning and environmental interaction.
- General Movement Assessment (GMA): The Prechtl General Movement Assessment is a widely recognized clinical tool for assessing neurological integrity in infants. Studies augmenting GMA with AI, even using single sensor modalities (like video), report classification performances competing around the 90% accuracy level. This suggests AI is approaching the proficiency of trained human assessors for specific, standardized assessments, potentially enabling wider screening.
- Sensor Fusion Advantage: Research indicates that combining data from multiple sensors (sensor fusion) significantly enhances accuracy. One study found that a three-sensor fusion approach achieved 94.5% accuracy in classifying infant motor patterns, outperforming any single modality evaluated. This points towards the future development of more robust multi-modal AI systems for comprehensive assessment.
- Key Movement Indicators: AI analysis has successfully identified specific movements as particularly meaningful. For instance, foot movements were found to be highly indicative of how infants interacted with and learned from a connected mobile, showing the most dramatic changes across experimental stages. Other research suggests that asymmetrical poses detected by AI while an infant is in a crib might correlate with higher risks for conditions like autism spectrum disorder (ASD). This demonstrates AI's capacity to pinpoint specific behavioral markers relevant to development.
Implications for Early Intervention
The ability of AI to provide objective, potentially continuous, and highly accurate developmental insights has profound implications for early intervention. Traditional screening schedules might occur at 9, 18, and 30 months, with specific ASD screening recommended at 18 and 24 months. However, AI-powered monitoring could facilitate much earlier identification of developmental differences, such as neuromotor challenges , potential autism markers , or cognitive delays in premature infants. Early detection is critical because it allows for the initiation of intervention services during crucial developmental windows, maximizing their effectiveness and improving long-term outcomes for children. AI tools could act as a preliminary screening mechanism, flagging children who might benefit from a more formal developmental evaluation by a specialist.
Examples of AI Monitoring Tools
Several innovative tools are emerging, leveraging AI for developmental monitoring:
- MAIJU Jumpsuit: Developed at the University of Helsinki, this smart jumpsuit integrates multiple sensors and uses AI algorithms to conduct reliable, objective assessments of infant motor milestones during free play at home, achieving precision comparable to WHO expert assessments.
- Early Markers App: This system utilizes smartphone video recordings and AI analysis to screen infants for motor delays and other developmental milestones, aiming to provide parents with real-time feedback and potentially reduce anxiety.
- AI for Neonatology: In Neonatal Intensive Care Units (NICUs), AI is being applied to analyze complex data streams including neuroimaging, vital parameters, and biosignals to aid in survival analysis, assess neurological development, and diagnose conditions like retinopathy of prematurity in vulnerable newborns.
- Motion-Based Classification Systems: Research demonstrates systems using wearable inertial sensors to collect day-long movement data, which AI classifiers then use to distinguish between typically developing infants and those at risk for developmental delays.
These examples illustrate the shift towards leveraging technology for more accessible and potentially continuous developmental surveillance. The move from subjective parental recall or brief clinical snapshots to objective, quantifiable data collection represents a significant advancement. AI's capacity to analyze non-verbal cues like intricate movement patterns offers a unique window into infant cognition and learning even before language fully develops. Furthermore, the potential use of accessible hardware like smartphones or relatively simple wearables suggests that AI-powered monitoring could eventually democratize early screening, making it more accessible across diverse socioeconomic and geographic contexts, potentially reducing health inequities.
Section 2: Enhancing Cognitive Growth with AI-Driven Education
The Promise of Personalization
Beyond monitoring physical development, AI is poised to reshape how children learn. Traditional educational models often follow a "one-size-fits-all" approach, which may not effectively cater to the diverse learning needs, paces, and styles present in any group of children. Personalized learning aims to remedy this by tailoring educational experiences to the individual. AI emerges as a powerful catalyst in this domain, offering the potential to deliver truly individualized instruction at scale, adapting dynamically to each child's unique learning journey.
Mechanisms of AI Adaptation
AI-driven educational tools employ various sophisticated mechanisms to achieve personalization and optimize learning:
- Adaptive Learning Paths: At the heart of many AI educational tools are algorithms that continuously analyze a student's performance. Factors like accuracy on questions, time taken to respond, and patterns of interaction with the material are used to dynamically adjust the difficulty level and sequence of subsequent content. If a child masters a concept quickly, the system introduces more challenging material; if they struggle, it might offer simpler explanations, break down tasks, or provide additional practice.
- Cognitive Load Management: Effective learning requires managing cognitive load – ensuring the mental effort demanded is appropriate. AI systems can infer a learner's cognitive state, perhaps through performance metrics or even potentially by analyzing neurophysiological data like EEG signals in research settings. Based on this inference, the system can adjust the complexity or presentation of information to prevent cognitive overload (which hinders learning) or under-stimulation (which leads to boredom), thereby optimizing learning efficacy.
- Real-Time Feedback: A significant advantage of AI tools is their ability to provide immediate and specific feedback. Instead of waiting for a teacher to grade an assignment, students can receive instant corrections or guidance as they work through problems, helping them understand mistakes and reinforce correct concepts promptly.
- Content Personalization and Interaction: AI can tailor not just the difficulty but also the type of content presented, potentially recommending supplementary videos, articles, or activities based on a learner's profile and interests. Techniques like Natural Language Processing (NLP) enable AI-powered tutors and chatbots to understand student queries and engage in natural-sounding dialogue, answering questions and providing instruction. Reinforcement learning algorithms allow systems to experiment and learn over time which instructional strategies are most effective for a particular student.
Evidence of Cognitive Benefits
Numerous studies have investigated the effectiveness of AI in education, providing evidence of tangible benefits and context for initial claims about cognitive gains:
- Improved Test Scores: A study involving the adaptive learning company Knewton found that students using its AI-powered program demonstrated a 62% improvement in test scores compared to peers in traditional learning environments. This suggests a substantial impact on academic achievement through personalized adaptation.
- Enhanced Math Performance: Mathematics, often a challenging subject, has been a focus area for AI interventions. One study reported that an intelligent tutoring system improved students' math learning performance by more than 20%. Another investigation found an AI system boosted fifth graders' math grades by 30% while simultaneously reducing their learning anxiety by 20%.
- Increased Learning Efficiency: AI appears to accelerate the learning process. An AI-based learning model was shown to increase students' learning efficiency by 20% on average. Furthermore, AI systems can provide significantly more feedback opportunities; one study noted that students using AI tutoring systems received 2.5 times more feedback than those in traditional classroom settings, allowing for quicker error correction.
- Higher Accuracy and Satisfaction: AI assistance can lead to greater mastery. An AI-assisted tutoring system reportedly increased the correctness of students' answers from 80% to 95%. Beyond performance, user experience also seems to improve, with one AI-based learning model associated with a 30% increase in student satisfaction.
- Critical Thinking and Problem-Solving Support: While requiring careful implementation, AI shows potential beyond rote memorization. It can assist in aspects of critical thinking, such as academic research and scrutinizing theories. AI tools can also foster problem-solving skills by providing adaptive challenges and scaffolding , although concerns about over-reliance persist.
Examples of AI Educational Tools
The market offers a growing array of AI-powered educational tools for children:
- Comprehensive Platforms: Tools like Khan Academy Kids provide personalized lessons across subjects like math and reading, incorporating AI-driven feedback. DreamBox Learning focuses on math, using AI to create dynamically adaptive learning paths.
- Subject-Specific Apps: Prodigy Math uses a game-based approach with adaptive AI challenges. Duolingo Kids employs AI for gamified language learning, adjusting lessons based on progress. Photomath uses AI to recognize math problems from images and provide step-by-step solutions.
- Interactive Tools: Osmo uniquely blends physical play objects with tablet-based AI technology for activities in math, spelling, and creativity. Educational robots like Roybi Robot use AI to engage children with personalized stories, games, and lessons.
- Coding/STEM: Platforms like CodeSpark Academy introduce foundational coding concepts through AI-powered activities suitable for young learners, even pre-readers.
- Assessment/Feedback Tools: Systems like Illumine's AI Assessment help educators refine observational notes into meaningful assessments. AI-powered writing assistants can offer real-time language corrections and suggestions.
- Tutoring Systems: AI tutors and chatbots are becoming increasingly sophisticated. Georgia Tech's Jill Watson chatbot successfully handled around 10,000 student inquiries per semester with 97% accuracy. Other AI chatbots have demonstrated 91% accuracy in providing personalized guidance.
The proliferation of these tools highlights the tangible ways AI is entering educational settings. The capacity for personalization and targeted support holds particular promise for addressing the diverse needs within any classroom, potentially helping to narrow achievement gaps, especially for students requiring specific accommodations or those in underserved communities. However, this promise is accompanied by important considerations. While AI demonstrably boosts efficiency and performance on measurable tasks , a critical tension exists. Over-reliance on these tools, particularly those that provide answers readily , might inadvertently hinder the development of deeper cognitive skills such as critical thinking, genuine creativity, and the ability to grapple productively with challenging problems. Striking a balance that leverages AI's strengths while preserving space for independent thought and exploration is essential. Furthermore, the very foundation of AI personalization – data – presents a dual-edged sword. Effective adaptation relies on collecting and analyzing extensive student data , creating unparalleled opportunities for tailoring education but simultaneously introducing significant risks related to data privacy, security, and the potential for algorithmic bias to perpetuate inequities. The pursuit of data-driven personalization must therefore be inextricably linked with robust ethical frameworks and safeguards.
Section 3: Supporting the Supporters: AI for Caregivers
The Caregiver Challenge
The demands placed on caregivers – whether parents juggling work and family or early childhood educators managing classrooms – are immense. High rates of burnout are prevalent, driven by factors including significant administrative workloads, emotional stress, long hours, and often inadequate compensation or support. Reports from organizations like the World Economic Forum highlight a broader "care crisis," where the infrastructure supporting childcare and eldercare is strained, impacting individual well-being and economic productivity. AI is emerging as a potential ally in addressing these challenges, offering tools to streamline tasks and provide much-needed support.
AI as an Efficiency Engine: Automating Tasks
One of the most immediate applications of AI for caregivers lies in its ability to automate or significantly speed up time-consuming administrative and documentation tasks. This frees up valuable time and mental energy for caregivers to focus on direct interaction and care.
- Documentation Automation:
- Charting & Clinical Notes: In healthcare contexts relevant to caregiving (e.g., for children with medical needs or eldercare), AI tools like Abridge and Guardoc can listen to conversations and automatically generate summaries or chart notes, potentially cutting documentation time significantly.
- Childcare Observations & Reports: AI platforms designed for ECEC settings, such as iCare AI, K-Buddy, and Illumine, assist educators in writing daily reports, documenting observations, and tracking learning milestones. These tools can suggest relevant keywords, analyze the complexity of notes, translate languages, ensure consistency, and link observations to specific learning frameworks (like EYLF).
- Lesson Planning: AI can serve as a "co-pilot" for educators. Tools like Brisk Teaching and K-Buddy help generate lesson plans, activity ideas, and curriculum materials, reducing planning time.
- Grading: AI-powered grading tools like Gradescope have been shown to reduce the time educators spend on grading by as much as 70%.
- Administrative Automation:
- Scheduling & Care Coordination: AI-enhanced smart calendar apps (e.g., Reclaim.ai) can help caregivers manage complex schedules involving appointments, therapies, and work responsibilities. Platforms like Wellthy use AI to offer customized care coordination tools and support, particularly valuable for family caregivers. Other care management platforms facilitate communication and planning among multiple caregivers and providers.
- Communication: AI can automate routine communications. Chatbots can handle frequently asked questions from parents. Platforms like Brightwheel , Illumine , and K-Buddy use AI to help generate personalized progress updates, newsletters, and daily reports for parents, streamlining communication and fostering engagement.
- Financial Management: For families managing care-related expenses or finances for individuals with disabilities, AI-powered budgeting apps like Mint and YNAB can assist with tracking income, categorizing expenses, and setting savings goals. Specialized tools like Carefull use AI and machine learning to monitor finances for aging loved ones, detecting potential fraud.
- Drafting Documents: Generative AI tools like ChatGPT can assist caregivers with writing-intensive tasks, such as drafting emails to teachers, creating templates for Letters of Intent for individuals with special needs, or outlining personalized care plans based on provided information.
AI as a Support System: Personalized Assistance
Beyond automating tasks, AI can offer more personalized forms of support, providing information, guidance, and resources tailored to the caregiver's specific situation.
- Personalized Information & Guidance:
- Care Plans: AI tools can help generate initial drafts or templates for personalized care plans by prompting users for relevant information about the care recipient's history, needs, and goals.
- Activity Ideas: AI can act as a creative partner, generating personalized activity ideas for children based on their age, interests, or developmental goals, or suggesting engaging activities for care recipients. Several apps like DoodleTale, Nookly, and Wondertales.io focus on AI-generated stories and activities.
- Targeted Educational Content: AI systems can assess a caregiver's knowledge gaps or needs and provide targeted educational resources or training materials relevant to their situation.
- Mental Health & Well-being: Recognizing the high stress levels associated with caregiving, AI tools are being developed to offer emotional support. Mental health chatbots like Tess provide customized, immediate support for family caregivers. Digital mental health platforms leveraging Cognitive Behavioral Therapy (CBT) principles offer accessible, self-paced programs for stress management and resilience building. AI models are also being explored to assess caregiver burnout risk.
- Accessing Resources: AI can help caregivers navigate complex systems. For example, AI might help map out the steps involved in accessing public assistance programs, understanding eligibility criteria, or planning for major transitions like moving from school to employment for individuals with special needs. AI tools can also assist in finding information about accessing benefits like paid family leave.
Impact on Burnout and Quality Time
The cumulative effect of AI-driven task automation and personalized support holds significant potential for reducing caregiver stress and burnout. By lessening the mental load associated with scheduling, documentation, and information seeking , and by automating time-consuming administrative tasks , AI can free up caregivers' cognitive resources and time. This reclaimed time is invaluable. It allows parents and educators to dedicate more energy to what truly matters: building relationships, engaging in meaningful interactions, providing responsive care, and fostering a positive environment for children's growth and development.
Examples of AI Caregiver Support Tools
The landscape of AI tools for caregivers is diverse and growing:
- Documentation/Admin: Abridge, Guardoc , Brisk Teaching , iCare AI , K-Buddy , Illumine , Gradescope.
- Coordination/Scheduling: Wellthy , Reclaim.ai.
- Financial: Carefull , Mint, YNAB.
- Personalized Support/Info: ChatGPT , Tess chatbot , The Cape (parenting insights) , story/activity generators (DoodleTale, Nookly, Wondertales.io, etc.).
- Communication: Brightwheel.
These tools illustrate AI's capacity to address both the practical, logistical challenges (the "work" of caregiving) and the informational and emotional support needs of caregivers. This dual approach is crucial, as burnout often stems from a combination of overwhelming tasks and feelings of isolation or lack of resources. The most promising applications frame AI not as a replacement, but as a "co-pilot" – an assistant that handles specific burdens, thereby enhancing the human caregiver's capacity. Because caregiving is fundamentally relational , technology that frees up time for connection, empathy, and nuanced interaction aligns best with the core values of care. Ultimately, supporting the well-being of caregivers through AI indirectly benefits children, as reduced caregiver stress and increased capacity contribute to higher quality interactions and a more nurturing environment essential for healthy development.
Section 4: Navigating the Future: Technology, Ethics, and the Human Touch
As AI continues to weave itself into the fabric of childcare, understanding the underlying technologies and navigating the complex ethical landscape becomes increasingly critical. Responsible innovation requires not only harnessing AI's potential but also proactively addressing its challenges and ensuring it serves, rather than supplants, human connection and well-being.
Underlying Technologies
Several core AI technologies power the applications discussed:
- Machine Learning (ML) / Deep Learning (DL): These are the workhorses behind many AI capabilities. ML algorithms learn patterns from data without being explicitly programmed for every task. Deep Learning, a subset of ML using neural networks with multiple layers, excels at complex pattern recognition, making it fundamental for analyzing infant movement data, assessing student performance in adaptive learning systems, and driving predictive analytics.
- Natural Language Processing (NLP): NLP enables computers to understand, interpret, and generate human language. This technology powers educational chatbots, AI assistants that summarize notes or draft communications, language learning applications that provide pronunciation feedback, and voice-controlled interfaces.
- Computer Vision: This field allows AI systems to "see" and interpret visual information from images or videos. It's used in analyzing infant movements from video recordings, potentially recognizing facial expressions or emotions (an area fraught with ethical debate), and monitoring interactions within a learning environment.
- Sensor Technology: The effectiveness of many AI monitoring systems depends on the quality of data gathered by sensors. This includes wearable devices with inertial measurement units (IMUs), 3D motion capture systems used in research, and even the cameras and microphones integrated into smartphones and tablets.
Ethical Considerations and Data Privacy
The deployment of these powerful technologies in childcare necessitates careful consideration of several critical ethical issues and robust data privacy measures:
- Data Privacy & Security: This is arguably the most significant concern, given the sensitivity of data collected from children, who represent a vulnerable population. Strict adherence to data protection regulations like the Children's Online Privacy Protection Act (COPPA) in the US and GDPR in the EU is essential. Best practices include:
- Data Minimization: Collecting only the data strictly necessary for the intended purpose.
- Transparency & Informed Consent: Clearly explaining what data is collected, how it's used and stored, and obtaining explicit, understandable consent from parents/guardians.
- Robust Security: Employing strong encryption, anonymization where possible, secure storage, and access controls to prevent breaches and unauthorized use.
- Algorithmic Bias & Fairness: AI systems learn from data, and if that data reflects existing societal biases (related to race, gender, socioeconomic status, language, etc.), the AI can perpetuate or even amplify those biases. This could lead to unfair outcomes in developmental assessments, biased educational recommendations, or inequitable access to resources. Mitigation requires using diverse and representative training data, actively auditing algorithms for bias, and designing systems with fairness as a core principle.
- Impact on Child Development: Concerns exist that excessive or inappropriate use of AI tools could negatively impact crucial aspects of child development. This includes potential hindrance of social skills development due to reduced peer interaction, dampening of creativity or critical thinking if AI provides answers too readily, weakening of emotional intelligence, and disruption of the essential bonding and attachment processes between children and caregivers. Ensuring a balance with screen time, prioritizing human interaction, promoting active rather than passive engagement, and demanding developmentally appropriate design are crucial safeguards.
- Transparency & Accountability: Many advanced AI models operate as "black boxes," making it difficult to understand precisely how they arrive at a decision or recommendation. This lack of transparency erodes trust and makes accountability challenging. While perfect explainability may not always be feasible, striving for interpretable models where possible and providing clear communication about AI system capabilities and limitations is vital.
- Autonomy & Manipulation: There are ethical concerns about AI, particularly systems designed to be persuasive or to recognize/respond to emotions, potentially manipulating children's choices, behaviors, or emotional states. Fostering digital literacy and critical thinking skills from an early age is important to empower children to navigate these technologies responsibly.
- Professional Ethics: For educators using AI documentation tools, there's an ethical responsibility to avoid misrepresenting AI-generated content as their own insights. Acknowledging the tool's contribution and maintaining professional judgment, grounded in relationships and pedagogical knowledge, is essential.
These ethical challenges are often interconnected. For instance, biased algorithms can worsen existing inequities , while privacy breaches undermine the trust needed for parents and educators to adopt beneficial technologies. The opacity of complex "black box" algorithms makes it harder to detect or correct bias. This highlights the need for a holistic ethical framework that addresses privacy, fairness, developmental impact, and transparency in concert. Furthermore, a tension exists between performance and accountability; the most powerful deep learning models often used for complex tasks like subtle developmental pattern detection are frequently the least interpretable. Finding a balance or developing better explainable AI (XAI) methods suitable for the sensitive context of childcare is an ongoing challenge. Finally, existing regulations often struggle to keep pace with AI advancements and may not fully address the unique vulnerabilities of young children. This points to a critical governance gap and the need for proactive, child-centric AI policies developed through collaboration among all stakeholders.
The Irreplaceable Human Element
Amidst the discussion of AI's capabilities, it is imperative to underscore the irreplaceable role of human connection in childcare. AI should be viewed as a tool to augment human capabilities, not replace the essential functions performed by parents, educators, and caregivers. Qualities like empathy, intuition, the ability to build deep trusting relationships, nuanced ethical judgment, and the capacity for responsive, attuned caregiving are uniquely human and fundamental to healthy child development. Technology can assist with tasks, provide data, and offer personalized pathways, but it cannot replicate the warmth, understanding, and complex social-emotional interactions that form the bedrock of quality childcare.
Conclusion: Embracing AI Responsibly in Childcare
Artificial intelligence is undeniably opening a new chapter in childcare, presenting transformative potential across developmental monitoring, personalized education, and caregiver support. From providing early warnings of developmental delays through sophisticated movement analysis to tailoring educational content with remarkable precision and alleviating the administrative burdens that contribute to caregiver burnout, AI offers tools that could significantly enhance the ECEC landscape.
However, this potential must be pursued with profound responsibility and ethical foresight. The integration of AI into the lives of young children demands unwavering prioritization of their well-being. This includes establishing robust safeguards for data privacy and security, actively working to identify and mitigate algorithmic bias to ensure fairness, critically evaluating the impact on holistic child development, and demanding transparency and accountability from AI systems and their developers. Most importantly, technology must always remain a tool in service of human connection, augmenting rather than replacing the vital roles of parents and educators.
The future of AI in childcare is not predetermined. Its ultimate impact will depend on the choices made today. By fostering ongoing research, encouraging critical evaluation of AI tools, and promoting collaboration between educators, parents, developers, and policymakers, it is possible to guide the development and deployment of AI in ways that are ethical, equitable, and truly beneficial. If navigated thoughtfully, AI can become a powerful ally, contributing positively to the support, growth, and flourishing of future generations.
FAQs About AI-Powered Childcare: Ushering in a New Era of Support, Safety & Smart Parenting
- Question:
What is AI-powered childcare? - Answer:
AI-powered childcare refers to the use of artificial intelligence technologies to support parents, caregivers, and childcare providers in ensuring children’s safety, development, and wellbeing. It includes tools like smart baby monitors, AI-based learning apps, personalized parenting recommendations, and real-time safety alerts, all designed to make parenting smarter and more responsive. - Question:
How can AI improve child safety at home? - Answer:
AI enhances child safety by using sensors, cameras, and machine learning to monitor environments in real time. Smart baby monitors, for instance, can detect unusual breathing patterns or movements, while AI home systems can alert parents if a child enters a restricted area or is in danger, offering peace of mind and quicker responses. - Question:
Can AI help parents with daily routines? - Answer:
Yes, AI can assist with scheduling, reminders, and customized routines based on a child’s age and needs. Apps powered by AI can suggest mealtimes, nap times, and learning activities, adapting based on behavior patterns to create healthier and more productive routines for both kids and parents. - Question:
Is AI-based parenting safe and reliable? - Answer:
AI tools for parenting are generally safe when sourced from trusted companies that prioritize data privacy and security. While AI can provide valuable insights and support, it should complement—not replace—human judgment. Parents should always be actively involved and use AI as a guide, not a substitute. - Question:
How does AI support children’s learning and development? - Answer:
AI-powered learning platforms analyze a child’s learning pace, strengths, and challenges to offer personalized educational experiences. These tools can suggest age-appropriate content, adjust difficulty levels in real-time, and even provide feedback to parents on developmental milestones.
sources :
scitechdaily.com
New AI Unlocks the Secrets of Infant Learning and Development
med.stanford.edu
News | Immunology | Stanford Medicine
pmc.ncbi.nlm.nih.gov
Challenging Cognitive Load Theory: The Role of Educational ...
pubmed.ncbi.nlm.nih.gov
Toward Predicting Infant Developmental Outcomes From Day-Long ...
researchgate.net
(PDF) Enhancing Early Childhood Cognitive Development via Mobile Game-Based Learning Applications: Insights and Practical Experiences - ResearchGate
researchgate.net
(PDF) Artificial Intelligence in Neuroeducation: A Systematic Review of AI Applications Aligned with Neuroscience Principles for Optimizing Learning Strategies - ResearchGate
stanmed.stanford.edu
How Stanford Medicine is capturing the AI moment
mdpi.com
AI in the Classroom: Insights from Educators on Usage, Challenges, and Mental Health
contemporarypediatrics.com
AI in pediatrics: Past, present, and future
pmc.ncbi.nlm.nih.gov
Artificial intelligence (AI) -integrated educational applications and college students' creativity and academic emotions
tandfonline.com
Full article: Critical thinking in the AI era: An exploration of EFL students' perceptions, benefits, and limitations - Taylor & Francis Online
pmc.ncbi.nlm.nih.gov
The past, current, and future of neonatal intensive care units with artificial intelligence: a systematic review
mdpi.com
New Era of Artificial Intelligence in Education: Towards a ... - MDPI
bernama.com
Rising Demand For Caregivers Highlights Long-term Care Economics - WEF - Bernama
profiles.stanford.edu
Dennis Wall - Stanford Profiles
jsser.org
Role of Artificial Intelligence in Enhancing Learning Outcomes of Pre-Service Social Studies Teachers Abdulaziz Al-Shammari1 &am
www3.weforum.org
www3.weforum.org
iejme.com
Artificial intelligence in mathematics education: A systematic literature review
weforum.org
The future of the care economy relies on collaboration and a 'care mindset'
ssir.org
How Investors Can Shape AI for the Benefit of Workers
eurekalert.org
AI predicts developmental paths in premature babies - EurekAlert!
unleash.ai
We're living in a caregiving crisis | UNLEASH
weforum.org
This AI helps parents and caregivers access paid leave - The World Economic Forum
sciencedaily.com
Innovative infant wearable uses artificial intelligence for at-home assessments of early motor development | ScienceDaily
specialneedsanswers.com
AI Tools Can Support Families Navigating Special Needs Care
cdc.gov
Developmental Monitoring and Screening - CDC
codeyoung.com
Best Interactive AI Learning Tools for Kids: Top Apps and Platforms to Get Started
fastercapital.com
Childcare staff Navigating Staff Burnout in Childcare: Strategies and Support - FasterCapital
researchgate.net
Artificial intelligence in child development monitoring: A systematic review on usage, outcomes and acceptance | Request PDF - ResearchGate
researchgate.net
The impact of AI enablement on students' personalized learning and countermeasures—A dialectical approach to thinking - ResearchGate
family-first.com
Caregiving Tools for Employers and Employees | Family First
pmc.ncbi.nlm.nih.gov
Focused review on artificial intelligence for disease detection in infants - PMC
claned.com
The Role of AI in Personalized Learning | Claned
pmc.ncbi.nlm.nih.gov
Artificial Intelligence Support for Informal Patient Caregivers: A Systematic Review - PMC
aicompetence.org
AI Education Apps Put To The Test: Which Ones Really Help?
mhealth.jmir.org
The Acceptability and Effectiveness of Web-Based Developmental Surveillance Programs: Rapid Review - JMIR mHealth and uHealth
learntolive.com
Managing Stress and Burnout When Caring for Others - Learn to Live
axonpark.com
How Effective is AI in Education? 10 Case Studies and Examples - Axon Park
pmc.ncbi.nlm.nih.gov
Sensing and Artificial Intelligent Maternal-Infant Health Care Systems: A Review - PMC
pmc.ncbi.nlm.nih.gov
Digital health interventions to support family caregivers: An updated systematic review
xyonix.com
Can AI Detect Developmental Delays at Home? with Bharath Modayur of Early Markers | Your AI Injection — Xyonix, AI Consulting & Custom Solutions
buddyxtheme.com
Best 10 AI Tools for Parenting: Enhance Your Child's Learning and Creativity
repository.cam.ac.uk
Perspectives on the impact of generative AI on early‐childhood development and education - University of Cambridge
icaresoftware.com
AI Tools (Coming Soon Starting with Journaling and Child Development) - iCare Software
illumine.app
AI in Early Childhood Education - The Complete Handbook - Illumine
mdpi.com
Leveraging AI in E-Learning: Personalized Learning and Adaptive Assessment through Cognitive Neuropsychology—A Systematic Analysis - MDPI
illumine.app
Illumine: AI Powered Childcare Management Software
respeecher.com
Empowering Education: AI and Personalization in Kids' Learning Apps - Respeecher
famly.co
AI in early childhood education | Famly
gsconlinepress.com
AI in education: A review of personalized learning and educational technology
digitaldefynd.com
10 ways AI can be used in early childhood education [2025] - DigitalDefynd
powerschool.com
The Ultimate Guide to AI in Education: Benefits, Challenges, & Real-World Uses
kinderm8.com.au
AI For Childcare is Here: The Incredible Benefits of K-Buddy - Kinder M8
community.trustcloud.ai
Data privacy and AI: ethical considerations and best practices - TrustCommunity
researchconnections.org
Innovating responsibly: Ethical considerations for AI in early childhood education
enrollify.org
Ethical Considerations For AI Use In Education - Enrollify
womentech.net
What Are the Ethical Considerations of Using Tech in Childcare and Child Development?
jetlearn.com
The Ethical Implications of AI: What Parents Should Consider - JetLearn
elearningindustry.com
Data Privacy In AI-Driven Learning And Ethical Considerations - eLearning Industry
forbes.com
Ethics And Compliance Are Vital When Applying AI Tools In HR - Forbes
cela.org.au
AI and documentation: What are the ethical considerations? - CELA
Comments
No comments yet. Be the first to leave a comment!